Over the past few years, artificial intelligence (AI) generate a huge interest in research, and it is now an ubiquitous concept when it comes to medical imaging. This term, sometimes a little overused, also rises false ideas or hopes of a medical revolution. Talking about deep learning, neural networks and image segmentation, Valéry Ozenne and Maxime Sermesant, both engineers and researchers at the Liryc institute, share their thought on the application of artificial intelligence in the cardiac imaging field.
What is artificial intelligence?
Artificial intelligence is a very broad subject that consists of implementing a set of computer techniques and theories aimed at stimulating or reproducing in machines certain features of human intelligence, such as reasoning or learning.
The medical sector is one of the most promising application for the use of AI. This has urged all the IT giants, from Google to Microsoft, via Facebook or IBM, with its famous Watson Health program, to early act and invest money.
Over time, AI has become very specialized in the medical field on automatic learning and deep learning. This process takes place in two stages:
- From a large database that is “learned” on a computer, we seek to establish statistical associations that can help analyze, predict, model. This learning phase requires a large amount of data and can be relatively long to complete.
- Then, it becomes possible to use this model to analyze new data and determine the characteristics or decisions required. This second step is extremely fast and very well suited to many daily life’ situations. This fast implementation mainly explains the success of AI in many fields (autonomous driving, video monitoring, radiology image,…).
Artificial intelligence serving a better medical imaging
AI is omnipresent in today scientific literature of medical imaging, especially since the development of new algorithms called convolutional neural networks[1]. The so-called convolutional approach makes it possible to go further in learning how to classify images or signals.
Indeed, to that day, AI is very useful in the field of imaging, in two areas: image classification and organ segmentation. Algorithms to classify images can help in diagnosis, by classifying an image into a particular category of pathology.
Algorithms for image segmentation[2] are commonly used on all types of imaging and on a daily basis at the University Hospital. This is how AI saves time for practitioners both in diagnosis and during interventions. It also has the advantage of bypassing certain biases related to the operator’s interpretation.
In cardiology, for example, AI nowadays makes it possible to segment the cardiac cavities of the right or left atrium.
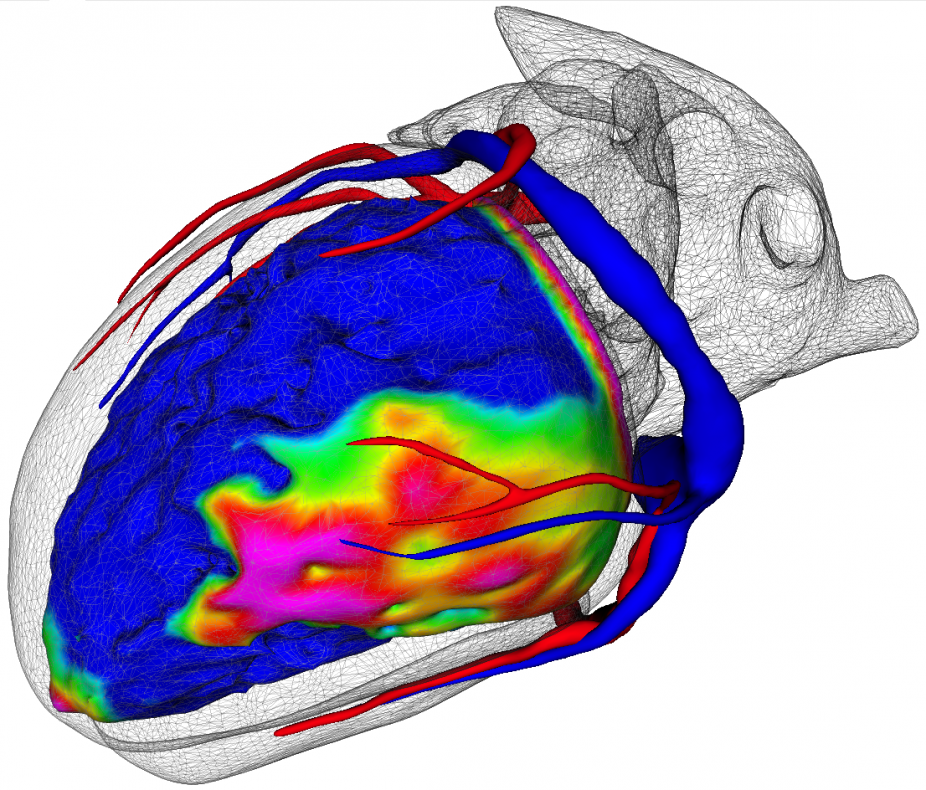
Artificial intelligence in imaging at Liryc
At the Liryc institute, AI is a cross-disciplinary thematic, mobilizing the collaboration of several research and clinical teams, with a specialized team at the interface, the multimodal data science team[3], formed in 2019.
On the imaging duties, Liryc’s teams use artificial intelligence to segment images. This involves segmenting reliefs, such as the ventricles’ outlines for example, or cardiac fibrosis, which is particularly diffuse. Segmentation tasks in cardiology is very complex because the heart is a moving muscle. AI makes it possible to simplify and accelerate the work of imaging teams, nowadays mainly in the preclinical stages, by facilitating the reading of images.
Learning segmentation methods can/will also be used on the interventional side by automatically segmenting the tissues treated during radiofrequency cardiac ablation procedures.
Another challenge of using AI at Liryc, lies in the exploitation of segmented data thanks to algorithms, which are then integrated into personalized electrophysiology models. The idea is to obtain a response specific to each patient. The modeling team, led by Edward Vigmond, uses artificial intelligence for this purpose. The algorithms describe the propagation of electrical activity in the heart muscle.
To be operational and robust, AI requires a large representative database, with enough specific cases, to optimize learning. This is possible at Liryc in particular thanks to the use of data recorded by the teams of the multimodal Music platform[4], which aggregates numerous anonymized clinical cases.
Between limits and future perspectives
Although AI is widely welcomed in practice, particularly with image segmentation, it has not yet proven its efficiency in terms of diagnosis and prognosis. The failure of IBM with Watson Health in an oncology study is a proof. However, the long-term objective is to be able to predict the evolution of a treatment and to advice which drug would be best suited. But to this day, many limitations prevent this projection, to a hyper-personalized medicine.
To do so, the computer must be able to justify its reasoning with full transparency, to be approved by practitioners. This transparency will also require the development of algorithms with high confidence indexes.
AI also raises the issue of data collection and anonymization, in the framework of strict regulatory policies and the need for infrastructure to manage data collection.
Others also point out a problem of bias in the development of artificial intelligence algorithms. The AI community is dominated by men, engineers, mostly white and belonging to the higher categories, who address specific problems. Between the biases of a human operator and the biases of an automatic operator, who could be the most objective? A question arises: can AI really be better than men?
The final word…
Regardless of the limits and challenges, the question of the capacity to create real added value for the medical sector is raised today, but AI has the merit of enhancing the value of the data collected, opening up an immense field of possibilities.
Like many institutions, Liryc is planning for the future with AI, with the ambition for AI to support the prediction of the risk of sudden cardiac death. Many parameters will have to be integrated also, subject to the interpretation of researchers and doctors upstream. There is a lot of development to be done, but with great potential.
[1] Invented in particular with the French researcher Yann Le Cun, “neural network” algorithms reproduce the convolution/abstraction processes of a brain. Usually, the algorithms weight the choices that arise and go for a middle path as the solution. However, the way the brain thinks is not linear. The brain aggregates several pieces of information and receives external stimuli; it sorts and chooses in a non-linear way. The neural network algorithm has succeded to have an approach, although simplified and mathematically optimized, less linear, closed to that of a human brain.
[2] In imaging, segmenting is an image processing operation that is equivalent to drawing the outlines of an organ, or a nodule for example.
[3] More information : https://www.ihu-liryc.fr/en/technology-for-health-cluster/p/music-/
[4] More information : https://www.ihu-liryc.fr/en/music/